News
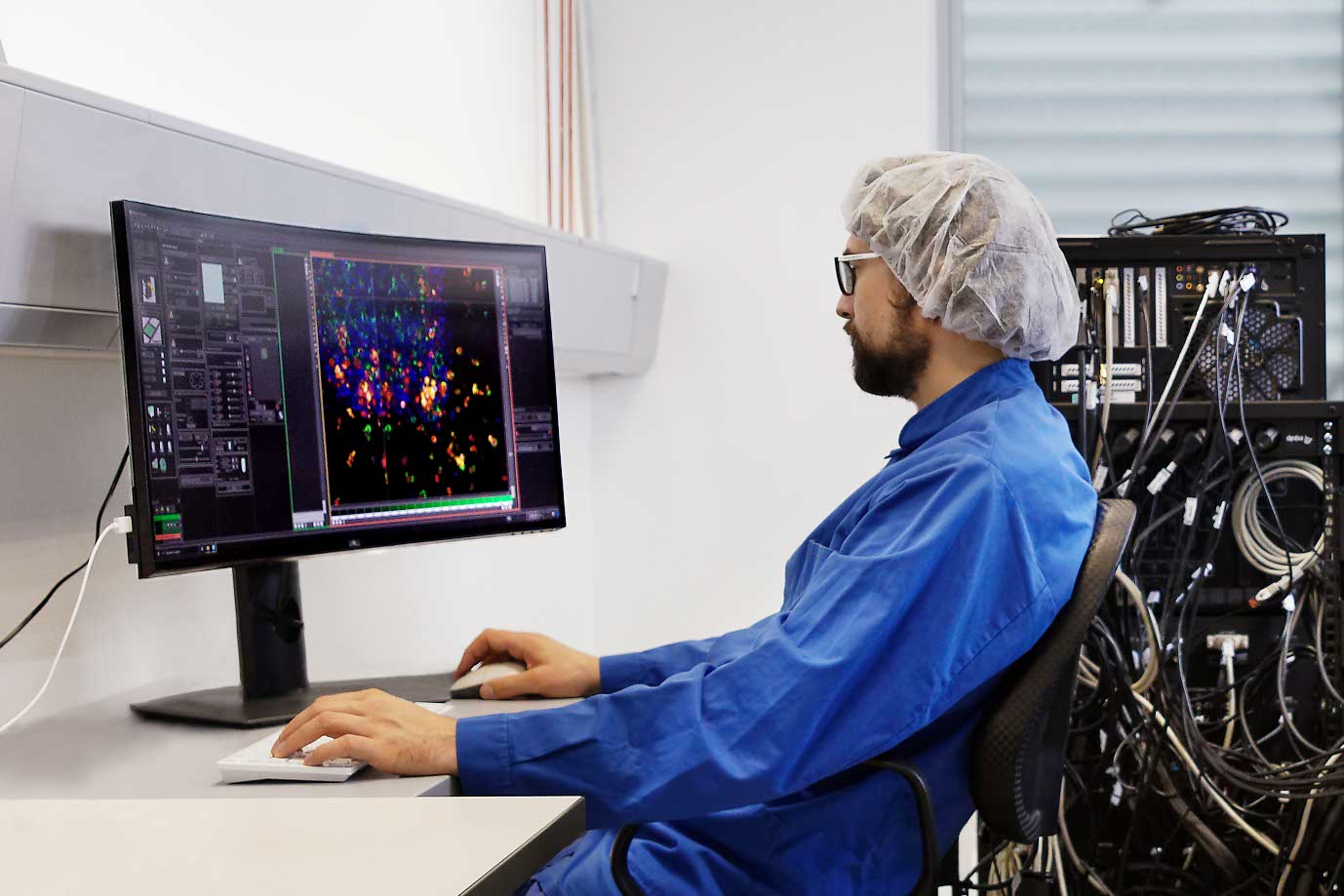
The future of cancer therapies
Researchers at the Werner Siemens Imaging Center are leading the way in the development of medical imaging techniques for cancer diagnostics. In a current project, they’re creating an AI-trained software program to analyse the massive amount of data generated by MRI and PET scanners—thus enabling groundbreaking insights into tumorous tissues.
Malignant tumours are complex: rather than consisting of uniform tissue, they’re often made up of very different tumorous areas and cell structures, which are themselves undergoing constant change. What’s more, each metastasis formed by these tumours is unique. This heterogeneity poses a challenge in treating many kinds of cancers, and the result is all too often a treatment that is ineffective—or a tumour that becomes resistant because it can’t be completely eradicated.
Fully characterising the diverse aspects of cancerous tumours is nearly impossible using today’s methods, in which doctors or researchers generally take a biopsy and then examine the tissue. “However,” says Bernd Pichler, head of the Werner Siemens Imaging Center (WSIC) in Tübingen, “a biopsy represents only a very small part of the tumour.” And because biopsy procedures are invasive, and sometimes dangerous, they can’t be conducted on a daily or weekly basis.
Smart data analysis
To better capture the heterogeneous nature of tumours, Pichler and his research team at WSIC are working to develop new, non-invasive diagnostic tools. They’ve made steady progress towards this aim, and last year saw the publication of several well-received papers on their projects, one of which applied an innovative approach to differentiating living, dead, necrotic and fibrotic tissues in a type of colon cancer.
For that study, the researchers examined tumours using a combined PET-MRI scanner. These techniques generate a massive amount of data, and to process all this information, the team created a machine learning software tool. In a first step, they fed the tool data from scans of some fifty mice with a colon cancer modelled on the kind that grows in humans; by processing the mouse data, the program learned how to distinguish between different tissue types.
In a second step, the team tested the software’s recognition capability on liver metastases in six colon cancer patients. “We were able to demonstrate that the algorithm translates very well to humans,” Bernd Pichler says. “And this is just a first example.” Indeed, the technology opens up previously unthinkable possibilities in tumour diagnostics: “It can also be used to examine and differentiate many other tumour properties.”
Now, the Tübingen researchers are aiming to distinguish aspects such as the various different stages in a cell cycle. Many tumour cells exist in what is known as a senescent state: although they no longer divide, they’re still alive and can “wake up” and trigger the growth of other tumour cells. Tracing them and finding the right moment to administer drugs that destroy them is of critical importance.
Personalised cancer treatments
The researchers believe that medical imaging techniques based on machine learning could be used to characterise the immune properties of various types of cancer cells as well as the traits of intercellular spaces—the extracellular matrix. First, however, special tracers (radio-labelled molecules) must be developed. After being introduced into a living organism, the tracers are absorbed by specific cells or used for targeted metabolic processes, depending on the type of molecule used. The tracers in the tissue are then rendered visible on scans generated by medical imaging techniques. As Bernd Pichler explains, “This helps us to better understand the microenvironment of tumours.”
It will also pave the way for new patient-specific therapeutic options. Last year, Bernd Pichler and his team published their vision for one such approach in a review article in Nature Reviews Cancer, a top-tier journal. In future, they write, advanced medical imaging techniques will help researchers identify a wide range of molecules, processes and properties in tumour tissues—thus laying the basis for personalised cancer therapies. “As just one example, we could study a tumour to find out how much the pH value should be adjusted to ensure that the chosen immunotherapy is effective,” says Pichler, who received the 2023 Gold Medal Award, the highest honour bestowed by the World Molecular Imaging Society.
Although it will still be many years before these visions become reality, the research groups at the WSIC are hard at work on their detection methods that are destined to revolutionise cancer treatment.